Table Of Content
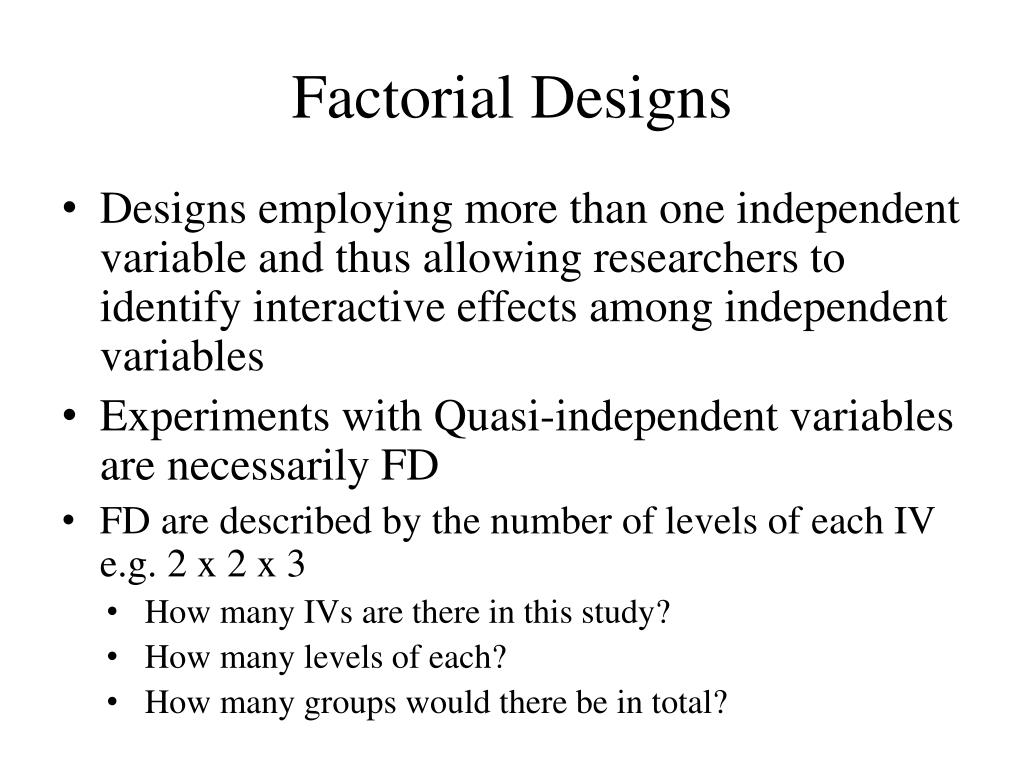
Other useful exploratory analysis tools for factorial experiments include main effects plots, interaction plots, Pareto plots, and a normal probability plot of the estimated effects. A factorial experiment allows for estimation of experimental error in two ways. The experiment can be replicated, or the sparsity-of-effects principle can often be exploited. Replication is more common for small experiments and is a very reliable way of assessing experimental error. When the number of factors is large (typically more than about 5 factors, but this does vary by application), replication of the design can become operationally difficult. In these cases, it is common to only run a single replicate of the design, and to assume that factor interactions of more than a certain order (say, between three or more factors) are negligible.
Fractional Factorial Experiments
Statistical modeling and optimization of heterogeneous Fenton-like removal of organic pollutant using fibrous catalysts ... - Nature.com
Statistical modeling and optimization of heterogeneous Fenton-like removal of organic pollutant using fibrous catalysts ....
Posted: Wed, 30 Sep 2020 07:00:00 GMT [source]
For information about the "Fold design" and "Add axial points", consult the "Help" menu. The main effect of a factor can be defined as the change produced as a result of a change in the level of the factor. Self-report measures involve asking participants to report their thoughts, feelings, or behaviors using questionnaires, surveys, or interviews. Replication involves conducting the same experiment with different samples or under different conditions to increase the reliability and validity of the results. Field experiments are conducted in naturalistic settings and allow for more realistic observations. However, because field experiments are not as controlled as laboratory experiments, they may be subject to more sources of error.
Minitab Example for Centrifugal Contactor Analysis
Investigators should consider using measures that would be sensitive to such effects. For instance, investigators might assess measures of burden (treatment fatigue) and determine if these are especially highly related to particular ICs or to an increasing number of ICs. Indeed, even without the use of special assessments, investigators might correlate the number of ICs a person receives (regardless of type) to outcomes. Factorial designs can pose challenges, but they offer important advantages that can offset such challenges. Of course, there is increased efficiency as investigators can screen more components at a reduced expenditure of resources. As the factorial design is primarily used for screening variables, only two levels are enough.
Minitab DOE Example
The first run (as specified by the random run order) should be performed at the low levels of A and C and the high levels of B and D. The following Yates algorithm table using the data from second two graphs of the main effects section was constructed. Besides the first row in the table, the main total effect value was 10 for factor A and 20 for factor B. However, since the value for B is larger, dosage has a larger effect on percentage of seizures than age.
Advances, challenges and opportunities in creating data for trustworthy AI
It might be found, for instance, that the impact of salary on worker productivity may be more pronounced for entry level as opposed to experienced employees. This type of analysis allows researchers to gain a deeper view of patterns that may emerge in the data set. The purpose of this page is to clarify some concepts, notation, and terminology related to factorial experimental designs, and to compare and contrast factorial experiments to randomized controlled trials (RCTs). In addition, the efficiency of a factorial experiment depends in part on the extent to which higher order interactions are not found. If interactions are found, and inferential statistics must be used to unpackage such interactions, such simple effects tests would require examining the effects of ICs in only subgroups of the sample.
Development of liposomes using formulation by design: Basics to recent advances - ScienceDirect.com
Development of liposomes using formulation by design: Basics to recent advances.
Posted: Fri, 11 Oct 2019 12:43:04 GMT [source]
The result of this study was that the participants high in hypochondriasis were better than those low in hypochondriasis at recalling the health-related words, but they were no better at recalling the non-health-related words. In sum, in a factorial experiment, the effects, relative effects, and statistical significance of ICs will likely change depending upon the number and types of components that co-occur in the experimental design. This arises, in part, from the fact that the effects of any given factor are defined by its average over the levels of the other factors in the experiment.
Lesson 5: Introduction to Factorial Designs
Once the design has been chosen, the "Factors...", "Options..." and "Results..." buttons become active in the "Create Factorial Designs" option menu. After the number of factors is chosen, click on the "Designs..." option to see the following menu. Belong to the A × B interaction; interaction is absent (additivity is present) if these expressions equal 0.[13][14] Additivity may be viewed as a kind of parallelism between factors, as illustrated in the Analysis section below. Frank Yates made significant contributions, particularly in the analysis of designs, by the Yates analysis. Cluster analysis is used to group similar cases or observations together based on similarities or differences in their characteristics.
Optimal experimental design
Note that here “treatment” is used to connote a set of intervention components (ICs); e.g., a particular type, dose, and duration of medication, type of counseling that is delivered for a particular number of sessions of a particular duration, and so on. The statistical analyses would reveal whether the experimental treatment “package” differs in effects from the usual care treatment. However, conducting an RCT that comprises ICs whose joint effects are unknown, poses clear risks. This is because research shows that the effectiveness of a IC can be substantially modulated by the other ICs with which it is used (Cook et al., 2016; Fraser et al., 2014; Schlam et al., 2016); i.e., they may interact.
Understanding Main Effects?
For example, when the main effect of keeping a food diary is estimated, the subjects in experimental conditions 3 and 4 are in the No level. Then, when the main effect of increasing physical activity is estimated, these same subjects are in the Yes level. This is one reason why factorial experiments are so efficient for examination of individual intervention components. Investigators may also wish to include measures in their factorial experiments that assess potential alternative explanations for their findings. We have discussed how the manipulation of multiple treatment factors might create unintended effects due to overall burden, inducement of optimism, apparent incompatibility of components or delivery routes, differential staff delivery, and so on.

So, for example, a 4×3 factorial design would involve two independent variables with four levels for one IV and three levels for the other IV. For instance, some interactions may be due to the overall burden due to subjects receiving large numbers of components. This might result in subadditive or negative interactions in which interventions produce less benefit, or even produce net decreases in benefit, when they co-occur with another intervention(s). This can pose interpretive challenges as it may be difficult to separate the effects of a component per se from the impact of burden.
One independent variable was disgust, which the researchers manipulated by testing participants in a clean room or a messy room. The other was private body consciousness, a participant variable which the researchers simply measured. Another example is a study by Halle Brown and colleagues in which participants were exposed to several words that they were later asked to recall (Brown, Kosslyn, Delamater, Fama, & Barsky, 1999)[1]. Some were negative health-related words (e.g., tumor, coronary), and others were not health related (e.g., election, geometry). The non-manipulated independent variable was whether participants were high or low in hypochondriasis (excessive concern with ordinary bodily symptoms).
Regression analysis is used to model the relationship between two or more variables in order to determine the strength and direction of the relationship. There are several types of regression analysis, including linear regression, logistic regression, and multiple regression. For example, suppose we wish to test the effects of a drug’s concentration in the range 0–100 ng/ml on the growth of cells. The cells will be grown with the drug in test tubes, arranged on a rack with four shelves.
ANOVA is a statistical technique used to compare means across two or more groups in order to determine whether there are significant differences between the groups. There are several types of ANOVA, including one-way ANOVA, two-way ANOVA, and repeated measures ANOVA. This involves systematically varying the order in which participants receive treatments or interventions in order to control for order effects. In this design, each participant is exposed to all of the different treatments or conditions, either in a random order or in a predetermined order. In this design, participants are randomly assigned to one of two or more groups, and each group is exposed to a different treatment or condition. Experimental design is a process of planning and conducting scientific experiments to investigate a hypothesis or research question.
In the remainder of this section, we will focus on between-subjects factorial designs only. Also, regardless of the design, the actual assignment of participants to conditions is typically done randomly. It is tempting to take advantage of the efficiency of the factorial experiment and use it to evaluate many components since power is unrelated to the number of factors, and therefore, a single experiment can be used to screen many components.
The numerical value of each of the numbers represents the number of levels of each independent variable. A 2 means that the independent variable has two levels, a 3 means that the independent variable has three levels, a 4 means it has four levels, etc. To illustrate, a 3 x 3 design has two independent variables, each with three levels, while a 2 x 2 x 2 design has three independent variables, each with two levels. To illustrate a 3 x 3 design has two independent variables, each with three levels, while a 2 x 2 x 2 design has three independent variables, each with two levels. Note that in both of these main effect comparisons, all of the experimental subjects are used. They are just rearranged to make each comparison, in a sort of “recycling” of subjects.
Thus, to obtain a precise estimate of β1, we want to place the concentration values as far apart as possible in order to stabilize the estimate. Assigning four or five units of each concentration to each shelf helps to reduce the confounding of drug and shelf effects. To understand the D-criterion (determinant), suppose we have a quadratic regression model2 with parameters β1 and β2 that relate the factor to the response (for simplicity, ignore β0, the intercept). Our estimates of these parameters, \(\hat \beta _1\) and \(\hat \beta _2\), will have error and, assuming the model error variance is known, the D-optimal design minimizes the area of the ellipse that defines the joint confidence interval for the parameters (Fig. 1). This area will include the true values of both β1 and β2 in 95% (or some other desired proportion) of repeated executions of the design, and its size and shape are a function of the data’s overall variance and the design.
No comments:
Post a Comment